top of page
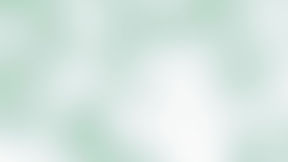
PET-MR image segmentation
In many applications, image segmentation is required at some point of the analysis, eg when assessing the texture of a tumor, when defining the biological tumor volume for treatment planning in radiotherapy, or when computing kinetic parameters. In PET, segmentation is always challenging due to the limited spatial resolution in PET images (from 4 to 8 mm depending on the PET system ). Guiding PET image segmentation by integrating higher resolution MR images (~1 mm for anatomical MRI), and conversely use of PET information to guide MR segmentation, is thus appealing. A possible strategy consists in using an initial tumor localization derived from PET data, which is then used to constrain a deformable model for accurately segmenting the tumor in high resolution images, as done previously for PET-CT data (see Figure). The latter scheme allows for many refinements such as performing tumor segmentation into parts by combining deformable models and fuzzy sets, or jointly exploiting the information contained in multi-protocol MRI scans using multi-phase and multi-channel level set techniques. Several aspects will be investigated to move forward in that research.
We will also investigate the use of structural knowledge and spatial reasoning for modeling and understanding the structure and evolution of tumors within their anatomical context from PET-MRI data.
Partners:
-
Hene Urien, PhD candidate
-
Isabelle Bloch, Professor at Telecom ParisTech, thesis advisor
-
Nicolas Rougon, Associate Professor at Telecom SudParis, thesis co-advisor, task leader
-
Irene Buvat, Senior Researcher at CEA-SHFJ, project coordinator, thesis co-advisor
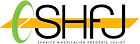


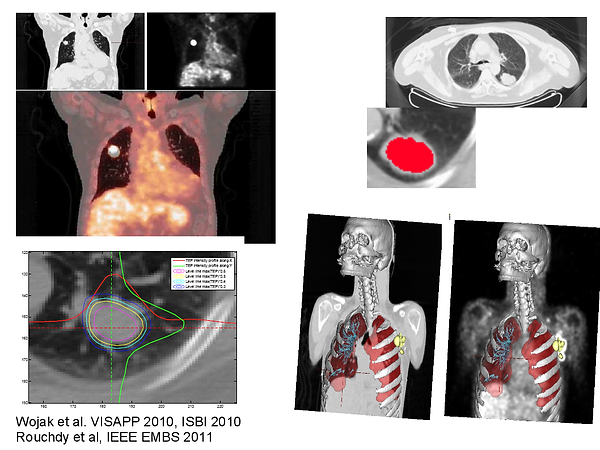
© Copyright
An example of anatomical
bottom of page